< Back to news 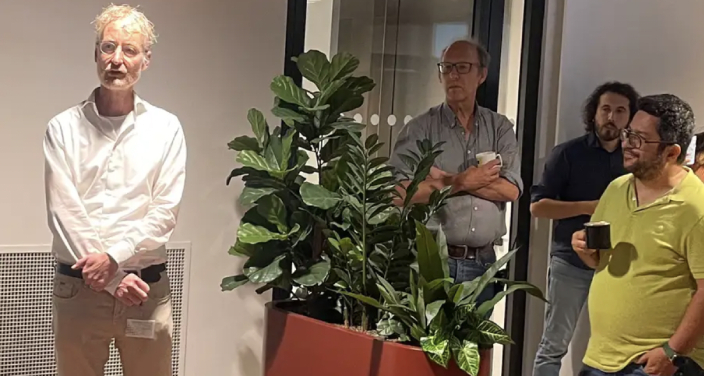
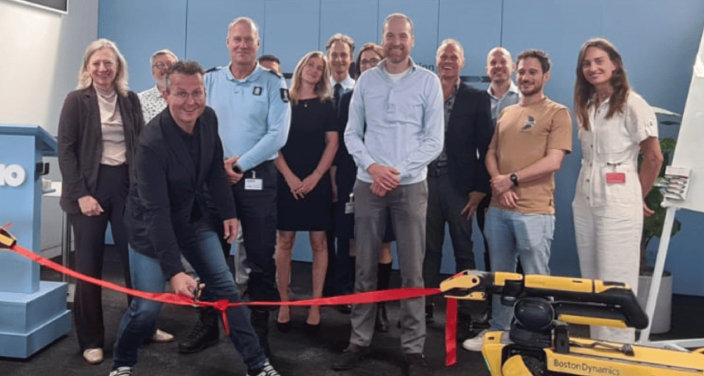
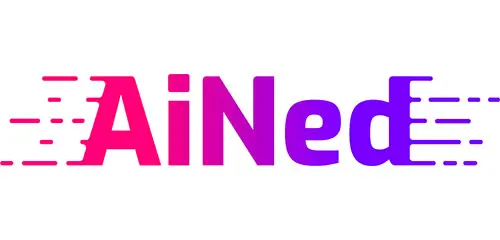
September 13, 2023
Explainable, Trustworthy, and Responsible AI in Image Processing
The technologies for image and video analysis and understanding have seen tremendous improvement in recent years. This is thanks to the emergence of modern deep learning network architectures (e.g., variants of RNNs such as LSTMs/GRUs, Generative Adversarial Networks, Transformers, Diffusion models) and learning paradigms (e.g., adversarial learning, deep reinforcement learning, contrastive learning) and the existence of large-scale datasets with ground-truth annotations that can assist the training of these architectures.
Moreover, there is a rapidly growing number of researchers interested in building methods that provide explanations about the working mechanisms and the decisions/predictions of deep neural networks. Already, there is notable progress in the field of pattern recognition (such as in deep learning architectures for image classification, object detection, semantic segmentation, and zero-shot learning), while there have been recent attempts at explaining the output of deep learning architectures dealing with video data (e.g. architectures for video event/activity recognition and classification, video captioning, and video summarization). While the success of deep learning models is tremendous, there are several challenges which still need to be addressed to adequately deploy these models in the real world.
Explainable: Models undergo significant variations in their accuracy which can be due to reasons such as variation in hyper-parameters or out-of-distribution images. These variations develop a sense of mistrust on the performance of the models and give the sense of being a black-box. Therefore, it is extremely important to decipher the knowledge these models are gaining to perform certain tasks such as face recognition or image classification.
Trustworthy: Deep learning models are sensitive to adversarial perturbations and natural noises. On top of that, the fairness of these models on different demographics in terms of face recognition and out-of-distribution images raises serious concerns. Therefore, it is important that the developed deep learning models are not only accurate but can effectively handle the variations in the images which occur through noises and images captured from persons from different demographics.
Responsible AI: The integration of AI technologies into more mainstream products and services needs to be accountable (AI developers need to be accountable for the actions and decisions of an AI-based system, especially a more autonomous one), inclusive (AI should consider all human races and experiences), reliable and safe (AI should perform as it was originally designed and for it to respond safely to new situations), fair (AI decisions do not discriminate or display a gender/race/sexual/religion bias toward a group or individual), transparent (about how the model was created e.g. which training data and algorithms it used, how it applied data transformations and other associated assets), and private and secure (how it secures personal information, applies different privacy protections, e.g., by randomizing data and adding noise to conceal personal information).
The goal of this Research Topic is the collection of novel machine learning models and datasets which can help in resolving the above-mentioned issues. This Research Topic accepts papers in the form of Original Research, Methods, Review, Hypothesis & Theory, Mini Review, Perspective, Case Report, Brief Research Report, Data Report on issues including but not limited to:
- Face recognition
- Development of novel machine learning and deep learning models
- Object Classification
- Fair AI
- XAI for image/video analysis and understanding
- Trustworthy AI
- Efficient and Effective AI
Explainable: Models undergo significant variations in their accuracy which can be due to reasons such as variation in hyper-parameters or out-of-distribution images. These variations develop a sense of mistrust on the performance of the models and give the sense of being a black-box. Therefore, it is extremely important to decipher the knowledge these models are gaining to perform certain tasks such as face recognition or image classification.
Trustworthy: Deep learning models are sensitive to adversarial perturbations and natural noises. On top of that, the fairness of these models on different demographics in terms of face recognition and out-of-distribution images raises serious concerns. Therefore, it is important that the developed deep learning models are not only accurate but can effectively handle the variations in the images which occur through noises and images captured from persons from different demographics.
Responsible AI: The integration of AI technologies into more mainstream products and services needs to be accountable (AI developers need to be accountable for the actions and decisions of an AI-based system, especially a more autonomous one), inclusive (AI should consider all human races and experiences), reliable and safe (AI should perform as it was originally designed and for it to respond safely to new situations), fair (AI decisions do not discriminate or display a gender/race/sexual/religion bias toward a group or individual), transparent (about how the model was created e.g. which training data and algorithms it used, how it applied data transformations and other associated assets), and private and secure (how it secures personal information, applies different privacy protections, e.g., by randomizing data and adding noise to conceal personal information).
The goal of this Research Topic is the collection of novel machine learning models and datasets which can help in resolving the above-mentioned issues. This Research Topic accepts papers in the form of Original Research, Methods, Review, Hypothesis & Theory, Mini Review, Perspective, Case Report, Brief Research Report, Data Report on issues including but not limited to:
- Face recognition
- Development of novel machine learning and deep learning models
- Object Classification
- Fair AI
- XAI for image/video analysis and understanding
- Trustworthy AI
- Efficient and Effective AI
Vergelijkbaar >
Similar news items
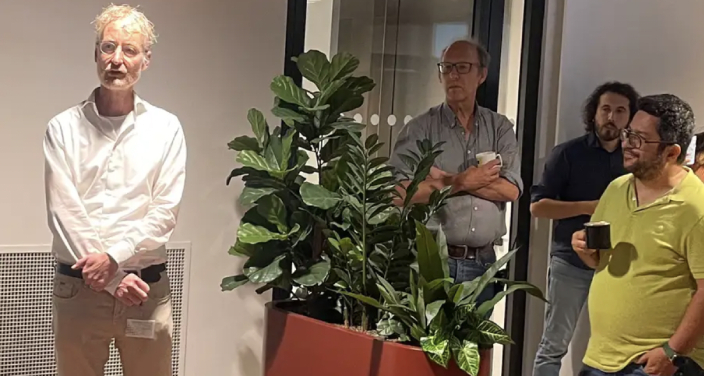
6 September 2024
CuspAI Introduces Itself at LAB42
On September 5, 2024, Max Welling and Chad Edwards, founders of CuspAI, presented their innovative company during the IvI coffee & cake gathering.
read more >
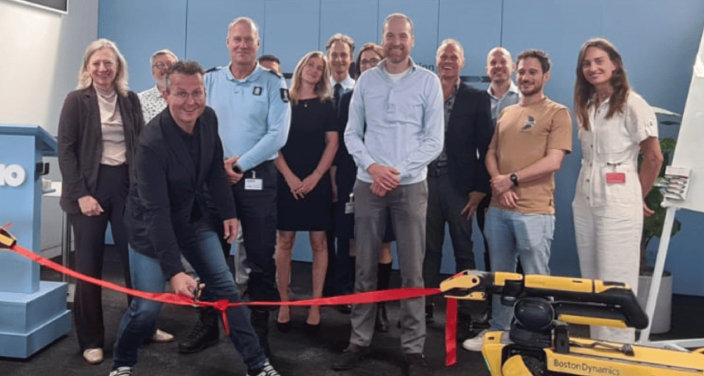
5 September 2024
Advanced AI for Surveillance Robots: A Collaborative Project by Dutch Institutions
A consortium of leading Dutch research institutions and government agencies, including TU Delft, the University of Amsterdam, TNO, and the Royal Netherlands Marechaussee, has launched an ambitious project aimed at developing advanced artificial intelligence (AI) for surveillance robots. Officially initiated on September 4, the OpenBots consortium focuses on creating AI systems designed to assist human security officers in various security settings.
read more >
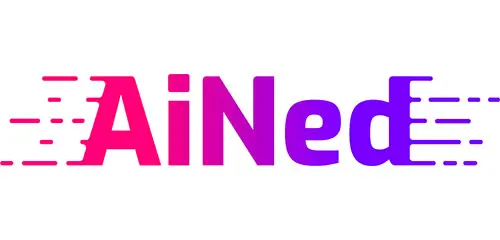
September 5, 2024
NeuroAI: Charlotte Frenkel explores the future of AI inspired by the human brain
With the award of an AiNed Fellowship grant, Dr. Charlotte Frenkel from TU Delft delves into neuromorphic computing, pioneering research aimed at creating energy-efficient and powerful AI systems inspired by the human brain. This research bridges AI and neuroscience to develop faster, more energy-efficient, and smarter computing systems.
read more >