< Back to news
To help overcome this problem, an international team of researchers, including multiple researchers from the University of Amsterdam’s Institute for Logic, Language and Computation (ILLC), has now published an Analysis in Nature Machine Intelligence. In the paper, they present a taxonomy for characterizing and understanding generalization research in NLP. The publication is the first result of the larger project GenBench, led by UvA-ILLC alumna Dieuwke Hupkes.
Lead author Mario Giulianelli (UvA-ILLC) comments: ‘The taxonomy we propose in our Analysis is based on an extensive literature review. We identified five axes along which generalization studies can differ: their main motivation, the type of generalization they aim to solve, the type of data shift they consider, the source by which this data shift originated, and the locus of the shift within the modern NLP modelling pipeline. Next, we used our taxonomy to classify over 700 experiments. We used these results to present an in-depth analysis that maps out the current state of generalization research in NLP and we make recommendations for which areas deserve attention in the future.’
NLP researchers interested in the topic of generalization can also visit the GenBench website. The website offers multiple tools for those interested in exploring and better understanding generalization studies, including an evolving survey, visualization tools and, soon, a generalization leaderboard. The first GenBench workshop will take place at the EMNLP 2023 conference, on December 6th. 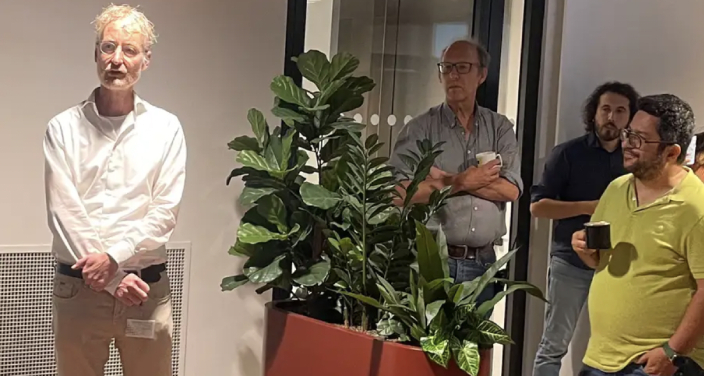
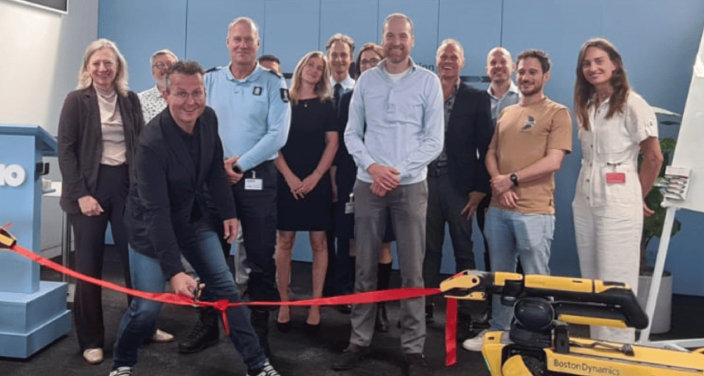
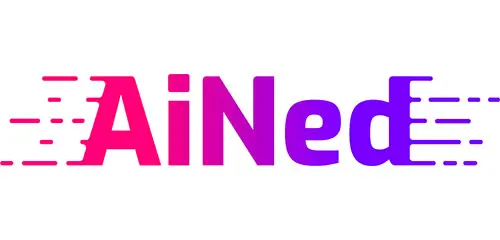
10 November 2023
New taxonomy aims to improve generalization research in NLP
Natural Language Processing—the subfield of computer science concerned with giving computers the ability to ‘understand’ and ‘generate’ human language—has taken a huge flight in the past decade.
The general public now knows about NLP because of the rise of tools like customer service chatbots on websites and ChatGPT. Meanwhile, research into how to further improve NLP models continues. In a paper in Nature Machine Intelligence an international team of scientists, including researchers of the University of Amsterdam, provide a framework to improve an aspect of NLP models called generalization.
The ability for NLP models to generalize well is one of the main desiderata of current NLP research. However, there is currently no consensus as to what ‘good generalization’ entails and how it should be evaluated. The rough definition is the ability to successfully transfer representations, knowledge and strategies from past experience to new experiences. So it can for instance be taken to mean that a model is able to apply predictions based on a certain data set to a new data set in a robust, reliable and fair way. But different researchers use different definitions. Also, there are currently no common standards to evaluate generalization. As a consequence, newly proposed NLP models are usually not systematically tested for their ability to generalize.
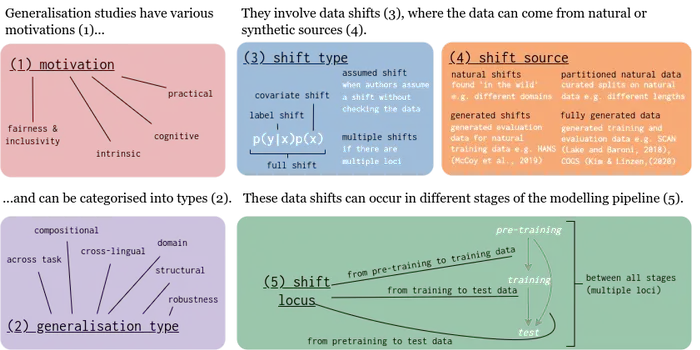
This infographic shows the five axes of the taxonomy. Credit: GenBench/UvA
GenBench
To help overcome this problem, an international team of researchers, including multiple researchers from the University of Amsterdam’s Institute for Logic, Language and Computation (ILLC), has now published an Analysis in Nature Machine Intelligence. In the paper, they present a taxonomy for characterizing and understanding generalization research in NLP. The publication is the first result of the larger project GenBench, led by UvA-ILLC alumna Dieuwke Hupkes.
Five axes
Lead author Mario Giulianelli (UvA-ILLC) comments: ‘The taxonomy we propose in our Analysis is based on an extensive literature review. We identified five axes along which generalization studies can differ: their main motivation, the type of generalization they aim to solve, the type of data shift they consider, the source by which this data shift originated, and the locus of the shift within the modern NLP modelling pipeline. Next, we used our taxonomy to classify over 700 experiments. We used these results to present an in-depth analysis that maps out the current state of generalization research in NLP and we make recommendations for which areas deserve attention in the future.’
Project website
NLP researchers interested in the topic of generalization can also visit the GenBench website. The website offers multiple tools for those interested in exploring and better understanding generalization studies, including an evolving survey, visualization tools and, soon, a generalization leaderboard. The first GenBench workshop will take place at the EMNLP 2023 conference, on December 6th.
This article was published on the website of the University of Amsterdam.
Vergelijkbaar >
Similar news items
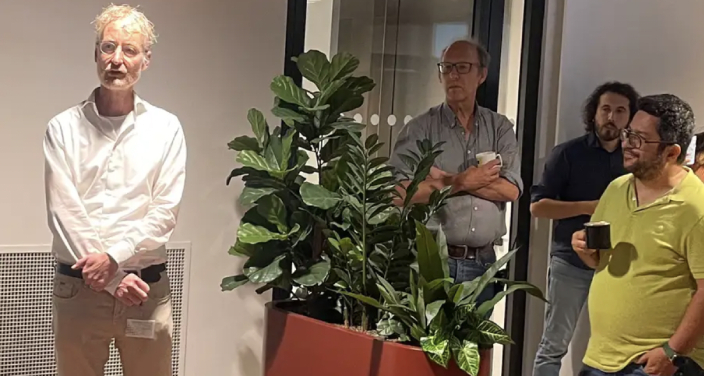
6 September 2024
CuspAI Introduces Itself at LAB42
On September 5, 2024, Max Welling and Chad Edwards, founders of CuspAI, presented their innovative company during the IvI coffee & cake gathering.
read more >
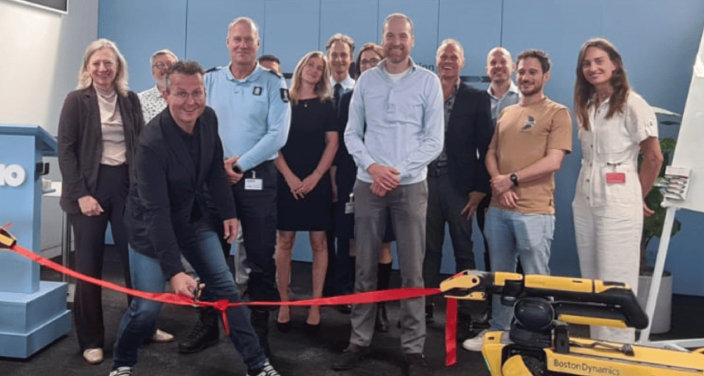
5 September 2024
Advanced AI for Surveillance Robots: A Collaborative Project by Dutch Institutions
A consortium of leading Dutch research institutions and government agencies, including TU Delft, the University of Amsterdam, TNO, and the Royal Netherlands Marechaussee, has launched an ambitious project aimed at developing advanced artificial intelligence (AI) for surveillance robots. Officially initiated on September 4, the OpenBots consortium focuses on creating AI systems designed to assist human security officers in various security settings.
read more >
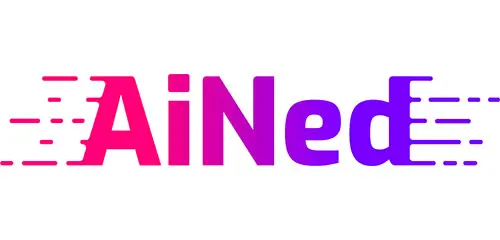
September 5, 2024
NeuroAI: Charlotte Frenkel explores the future of AI inspired by the human brain
With the award of an AiNed Fellowship grant, Dr. Charlotte Frenkel from TU Delft delves into neuromorphic computing, pioneering research aimed at creating energy-efficient and powerful AI systems inspired by the human brain. This research bridges AI and neuroscience to develop faster, more energy-efficient, and smarter computing systems.
read more >